Note
Go to the end to download the full example code or to run this example in your browser via Binder
Track solidification of a metallic alloy#
In this example, we identify and track the solid-liquid (S-L) interface in a nickel-based alloy undergoing solidification. Tracking the solidification over time enables the calculation of the solidification velocity. This is important to characterize the solidified structure of the sample and will be used to inform research into additive manufacturing of metals. The image sequence was obtained by the Center for Advanced Non-Ferrous Structural Alloys (CANFSA) using synchrotron x-radiography at the Advanced Photon Source (APS) at Argonne National Laboratory (ANL). This analysis was first presented at a conference [1].
import matplotlib.pyplot as plt
import numpy as np
import pandas as pd
import plotly.express as px
import plotly.io
from skimage import filters, measure, restoration
from skimage.data import nickel_solidification
image_sequence = nickel_solidification()
y0, y1, x0, x1 = 0, 180, 100, 330
image_sequence = image_sequence[:, y0:y1, x0:x1]
print(f'shape: {image_sequence.shape}')
shape: (11, 180, 230)
The dataset is a 2D image stack with 11 frames (time points). We visualize and analyze it in a workflow where the first image processing steps are performed on the entire three-dimensional dataset (i.e., across space and time), such that the removal of localized, transient noise is favored as opposed to that of physical features (e.g., bubbles, splatters, etc.), which exist in roughly the same position from one frame to the next.
fig = px.imshow(
image_sequence,
animation_frame=0,
binary_string=True,
labels={'animation_frame': 'time point'},
)
plotly.io.show(fig)
Compute image deltas#
Let us first apply a Gaussian low-pass filter in order to smooth the images and reduce noise. Next, we compute the image deltas, i.e., the sequence of differences between two consecutive frames. To do this, we subtract the image sequence ending at the second-to-last frame from the image sequence starting at the second frame.
smoothed = filters.gaussian(image_sequence)
image_deltas = smoothed[1:, :, :] - smoothed[:-1, :, :]
fig = px.imshow(
image_deltas,
animation_frame=0,
binary_string=True,
labels={'animation_frame': 'time point'},
)
plotly.io.show(fig)
Clip lowest and highest intensities#
We now calculate the 5th and 95th percentile intensities of image_deltas
:
We want to clip the intensities which lie below the 5th percentile
intensity and above the 95th percentile intensity, while also rescaling
the intensity values to [0, 1].
p_low, p_high = np.percentile(image_deltas, [5, 95])
clipped = image_deltas - p_low
clipped[clipped < 0.0] = 0.0
clipped = clipped / p_high
clipped[clipped > 1.0] = 1.0
fig = px.imshow(
clipped,
animation_frame=0,
binary_string=True,
labels={'animation_frame': 'time point'},
)
plotly.io.show(fig)
Invert and denoise#
We invert the clipped
images so the regions of highest intensity
will include the region we are interested in tracking (i.e., the
S-L interface). Then, we apply a total variation denoising filter to reduce
noise beyond the interface.
inverted = 1 - clipped
denoised = restoration.denoise_tv_chambolle(inverted, weight=0.15)
fig = px.imshow(
denoised,
animation_frame=0,
binary_string=True,
labels={'animation_frame': 'time point'},
)
plotly.io.show(fig)
Binarize#
Our next step is to create binary images, splitting the images into foreground and background: We want the S-L interface to be the most prominent feature in the foreground of each binary image, so that it can eventually be separated from the rest of the image.
We need
a threshold value thresh_val
to create our binary images, binarized
.
This can be set manually, but we shall use an automated minimum threshold
method from the filters
submodule of scikit-image (there are other
methods that may work better for different applications).
thresh_val = filters.threshold_minimum(denoised)
binarized = denoised > thresh_val
fig = px.imshow(
binarized,
animation_frame=0,
binary_string=True,
labels={'animation_frame': 'time point'},
)
plotly.io.show(fig)
Select largest region#
In our binary images, the S-L interface appears as the largest region of connected pixels. For this step of the workflow, we will operate on each 2D image separately, as opposed to the entire 3D dataset, because we are interested in a single moment in time for each region.
We apply skimage.measure.label()
on the binary images so that each
region has its own label. Then, we select the largest region in each image
by computing region properties, including the area
property, and
sorting by area
values. Function
skimage.measure.regionprops_table()
returns a table of region
properties which can be read into a Pandas DataFrame
.
To begin with, let us consider the first image delta at this stage of the
workflow, binarized[0, :, :]
.
labeled_0 = measure.label(binarized[0, :, :])
props_0 = measure.regionprops_table(labeled_0, properties=('label', 'area', 'bbox'))
props_0_df = pd.DataFrame(props_0)
props_0_df = props_0_df.sort_values('area', ascending=False)
# Show top five rows
props_0_df.head()
We can thus select the largest region by matching its label with the one found in the first row of the above (sorted) table. Let us visualize it, along with its bounding box (bbox) in red.
We can see how the lower bounds of the box align with the bottom of the S-L interface by overlaying the same bounding box onto the 0th raw image. This bounding box was calculated from the image delta between the 0th and 1st images, but the bottom-most region of the box corresponds to the location of the interface earlier in time (0th image) because the interface is moving upward.
fig = px.imshow(image_sequence[0, :, :], binary_string=True)
fig.add_shape(type='rect', x0=minc, y0=minr, x1=maxc, y1=maxr, line=dict(color='Red'))
plotly.io.show(fig)
We are now ready to perform this selection for all image deltas in the sequence. We shall also store the bbox information, which will be used to track the position of the S-L interface.
largest_region = np.empty_like(binarized)
bboxes = []
for i in range(binarized.shape[0]):
labeled = measure.label(binarized[i, :, :])
props = measure.regionprops_table(labeled, properties=('label', 'area', 'bbox'))
props_df = pd.DataFrame(props)
props_df = props_df.sort_values('area', ascending=False)
largest_region[i, :, :] = labeled == props_df.iloc[0]['label']
bboxes.append([props_df.iloc[0][f'bbox-{i}'] for i in range(4)])
fig = px.imshow(
largest_region,
animation_frame=0,
binary_string=True,
labels={'animation_frame': 'time point'},
)
plotly.io.show(fig)
Plot interface location over time#
The final step in this analysis is to plot the location of the S-L
interfaces over time. This can be achieved by plotting maxr
(third element in bbox)
over time since this value shows the y location of the bottom of
the interface. The pixel size in this experiment was 1.93 microns
and the framerate was 80,000 frames per second, so these values
are used to convert pixels and image number to physical units.
We calculate the average solidfication velocity by fitting a linear
polynomial to the scatter plot. The velocity is the first-order coefficient.
ums_per_pixel = 1.93
fps = 80000
interface_y_um = [ums_per_pixel * bbox[2] for bbox in bboxes]
time_us = 1e6 / fps * np.arange(len(interface_y_um))
fig, ax = plt.subplots(dpi=100)
ax.scatter(time_us, interface_y_um)
c0, c1 = np.polynomial.polynomial.polyfit(time_us, interface_y_um, 1)
ax.plot(time_us, c1 * time_us + c0, label=f'Velocity: {abs(round(c1, 3))} m/s')
ax.set_title('S-L interface location vs. time')
ax.set_ylabel(r'Location ($\mu$m)')
ax.set_xlabel(r'Time ($\mu$s)')
plt.show()
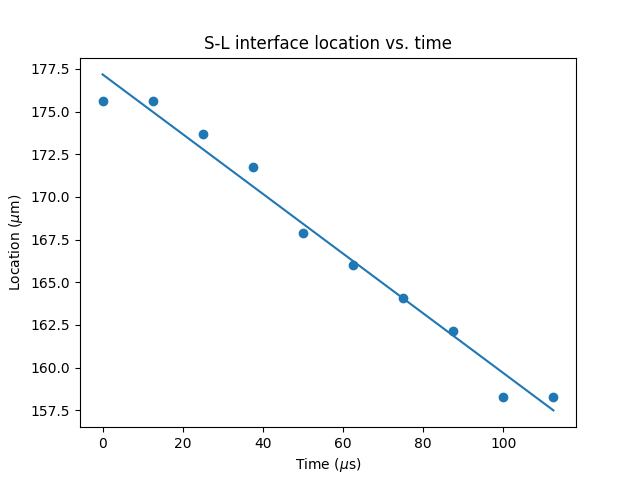
Total running time of the script: (0 minutes 2.747 seconds)